Discover how to fine-tune and train a Sentence Transformers model for sentence similarity search by harnessing the power of vector embeddings.
Introduction
Sentence Transformers is a widely recognized Python module for training or fine-tuning state-of-the-art text embedding models. In the realm of large language models (LLMs), embedding plays a crucial role, as it significantly enhances the performance of tasks such as similarity search when tailored to specific datasets.
Recently, Hugging Face released version 3.0.0 of Sentence Transformers, which simplifies training, logging, and evaluation processes. In this article, we will explore how to train and fine-tune a Sentence Transformer model using our data.
Embeddings for Similarity Search
Embedding is the process of converting text into fixed-size vector representations (floating-point numbers) that capture the semantic meaning of the text in relation to other words. How can this be used for similarity search? In similarity search, we embed queries into a vector database. When a user submits a query, we need to find similar queries in the database.
First, convert all textual data into fixed-size vector embeddings and store them in a vector database. Next, accept a query from the user and convert it into an embedding as well. Then, find similar search terms or keywords from the user query within the vector database and retrieve those embeddings that are closest. Is it simple? Yes, but to search for the closest embeddings, we need to use distance-based algorithms such as Cosine Similarity, Manhattan Distance, or Euclidean Distance.
What is SBERT?
SBERT (Sentence-BERT) is a specialized type of sentence transformer model tailored for efficient sentence processing and comparison. It employs a Siamese network architecture, utilizing identical BERT models to process sentence pairs independently. Additionally, SBERT utilizes mean pooling on the final output layer to generate high-quality sentence embeddings. For a comprehensive understanding of SBERT, I recommend referring to the detailed article.
Installation and setup
You can either use online notebooks such as Google Colab. I have also covered how to execute training code from script. For Google Colab, set your runtime environment to T4 GPU hardware.
Install necessary dependencies.
!pip install -U "sentence-transformers[train]" accelerate datasets
Import dependencies
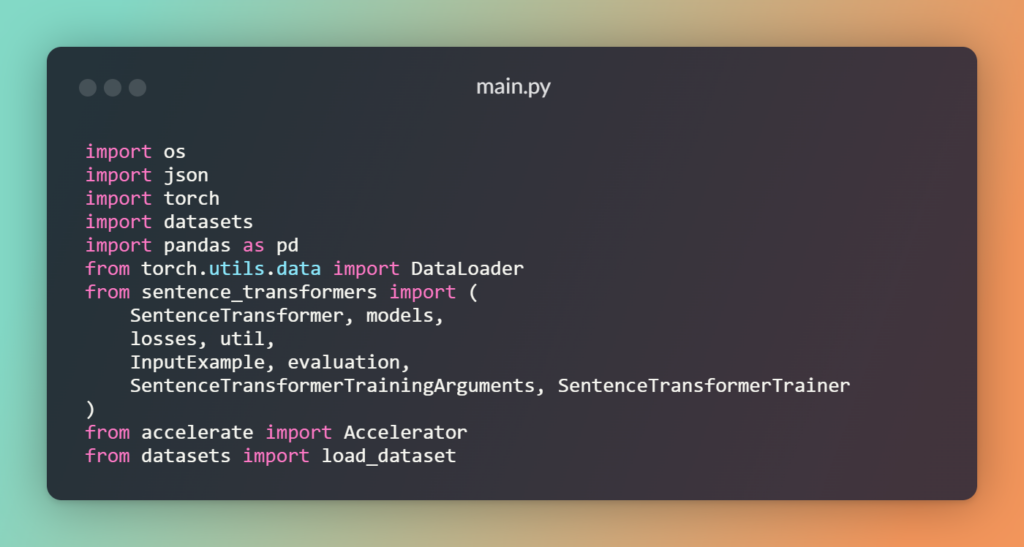
For this blog post, I am using Glue STS-B data and model sentence-transformers/all-MiniLM-L6-v2
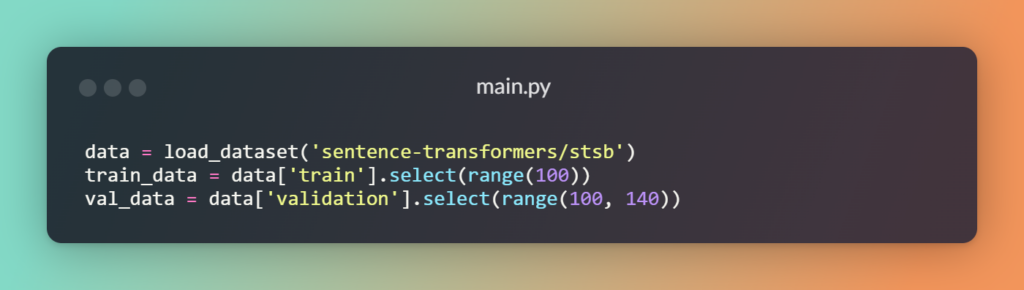
Let’s see random sample data from train data
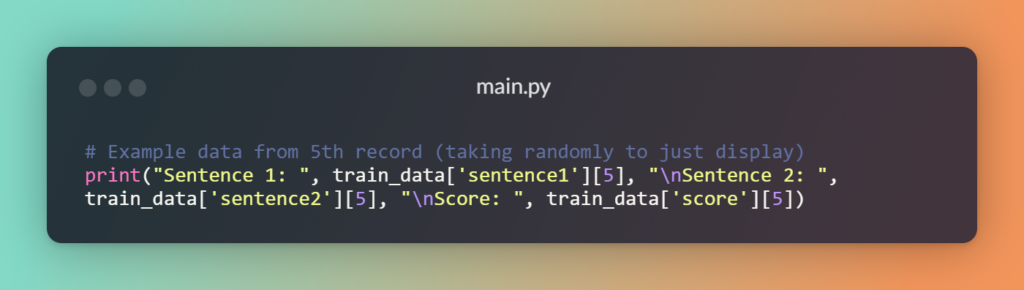
Output:
Sentence 1: Some men are fighting. Sentence 2: Two men are fighting. Score: 0.85
This will be the format of our data: ‘sentence1’, ‘sentence2’, and ‘score’. The ‘score’ represents the degree of closeness or similarity between the two sentences. In cases where a label score is unavailable, you simply need to modify the loss function and evaluator accordingly.
Training SBERT
This is recommended way to train SBERT model form SBERT official site.

To train the SBERT model, you need to encapsulate the model building, evaluator, and training processes within the main()
function, See this discussion.
Training Code:
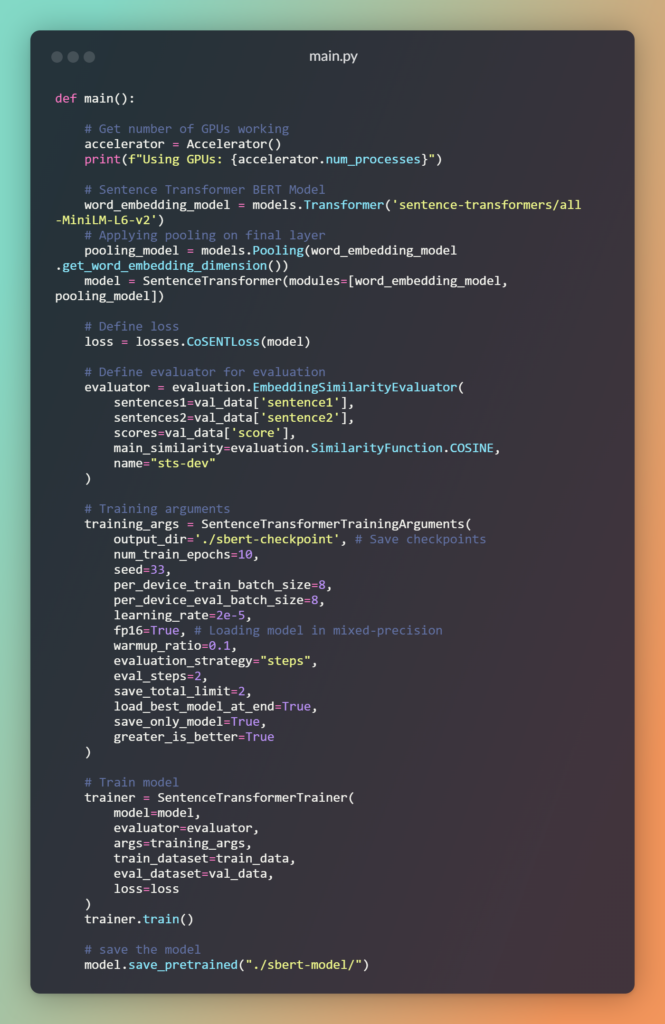
Now, let’s understand each component inside main()
function step by step,
- Define the
Accelerator()
to determine the number of GPUs available on the current machine. - Load the Sentence Transformers model from the HuggingFace repository and extract the word embedding dimension using mean pooling. Add a mean pooling layer after the SBERT model as output.
- Define the Loss function, such as
CoSENTLoss()
, to calculate the model’s loss based on float similarity scores. Choose the appropriate loss function from SBERT’s options based on your data and labels. Refer to the Loss Overview in the Sentence Transformers documentation. - Use the
Evaluator()
class provided by Sentence Transformers to calculate the evaluation loss during training and obtain specific metrics. Choose the appropriate evaluator, such asEmbeddingSimilarityEvaluator()
, based on your data and use case. Refer to this table for available options. - Specify training arguments, such as the output directory for storing checkpoints, batch size per device (CPU/GPU), number of training epochs, learning rate, float16 precision for model loading, evaluation steps, etc., using the
SentenceTransformerTrainer
class which is indirectly inherited from transformersTrainingArguments
. - Train the model using the
SentenceTransformerTrainer
class by defining the training and validation data, optionally including an evaluator, specifying training arguments, and defining the loss function. Initiate training by calling thetrain()
method. Perform training and save the model further.
Various Methods for Training SBERT
After defining the main()
function, simply call it to initiate the model training process. There are several ways to do this:
For Single GPU:
- If you are running code in Google Colab free version with T4 GPU then just create a new cell and call function:
main()
- If you are running your code in Python script, then just run a python command in the terminal:
python main.py
.
For Multi-GPU:
HuggingFace transformer supports DistributedDataParallel (DDP) training to perform distributed parallel training on multiple GPU or in multiple machines. Read this article to understand how DDP works.
- If you are running your code in colab or any notebook which contains multi-gpu, then:
from accelerator import notebook_launcher
notebook_launcher(main, num_processes=2)
By running above code in a separate will run your code in multi-gpu.
- For Python Script:
accelerate launch –multi-gpu –num_processes=2 main.py
These are some common ways to run a script or notebook for SBERT training.
Test the Model
After training the model, we can reload it and perform inference testing. For instance, if we have a list of product names and users input search terms, our goal is to identify the most similar product names along with a score.
Having trained our embedding model on sentence similarity data using similarity scores as labels, it will now improve the embeddings.
Here is the sample list of product name which we are using for embedding data:
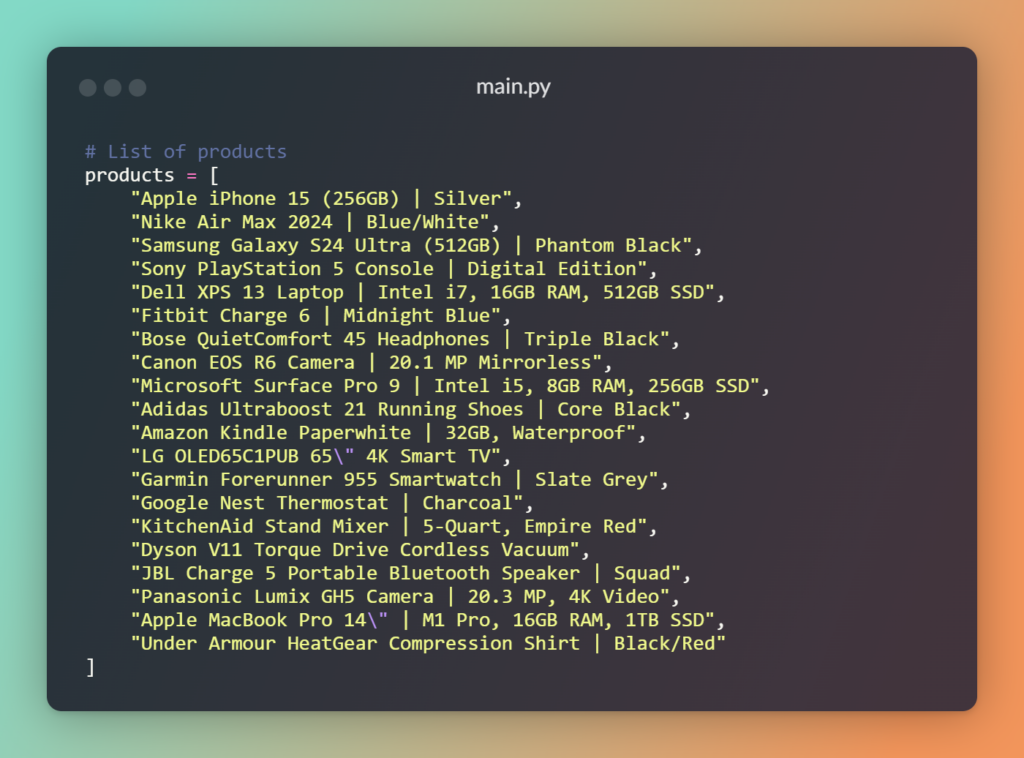
Next, load our fine-tuned SBERT model and convert product names into vector embeddings:
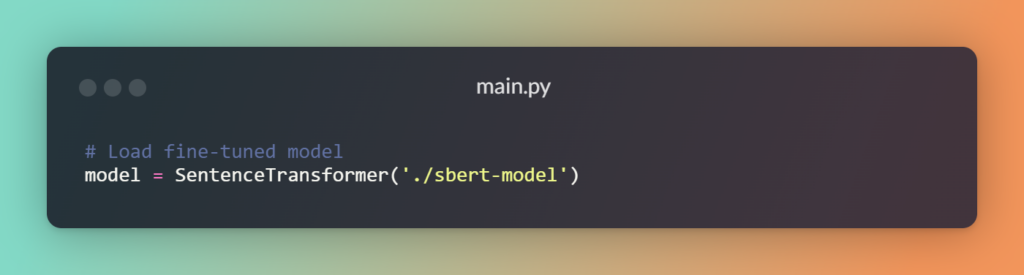
To convert product names into embeddings, we’ll utilize the GPU and convert them into tensors. You can do so using the following code:
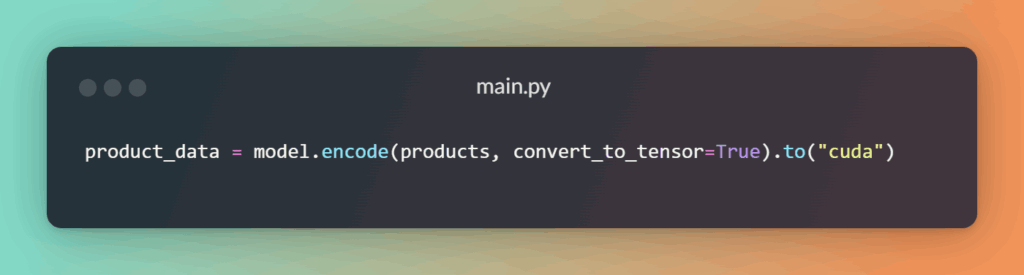
By converting embeddings to CUDA, we leverage GPU computational support (dtype=torch.float32); otherwise, if CPU is selected, it defaults to (dtype=float32).
This ‘product_data’ serves as our vector database, now stored in memory. Alternatively, you can utilize vector databases like Qdrant, Pinecone, Chroma, etc.
Lastly, create a function that accepts a user query from the terminal or as user input and returns the top products along with their Cosine-Similarity scores.
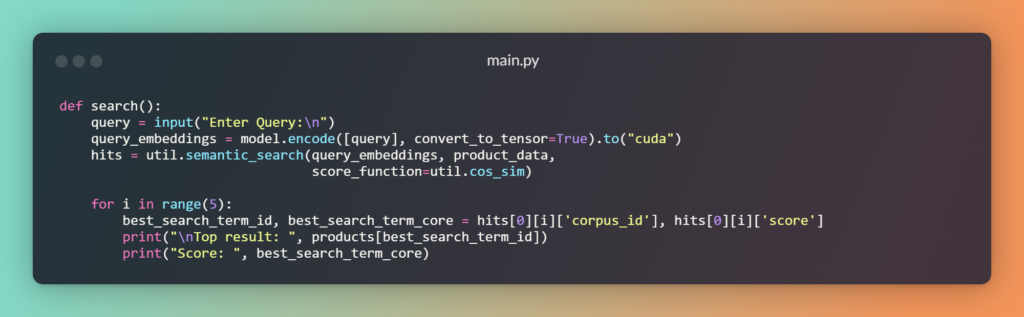
Test run:
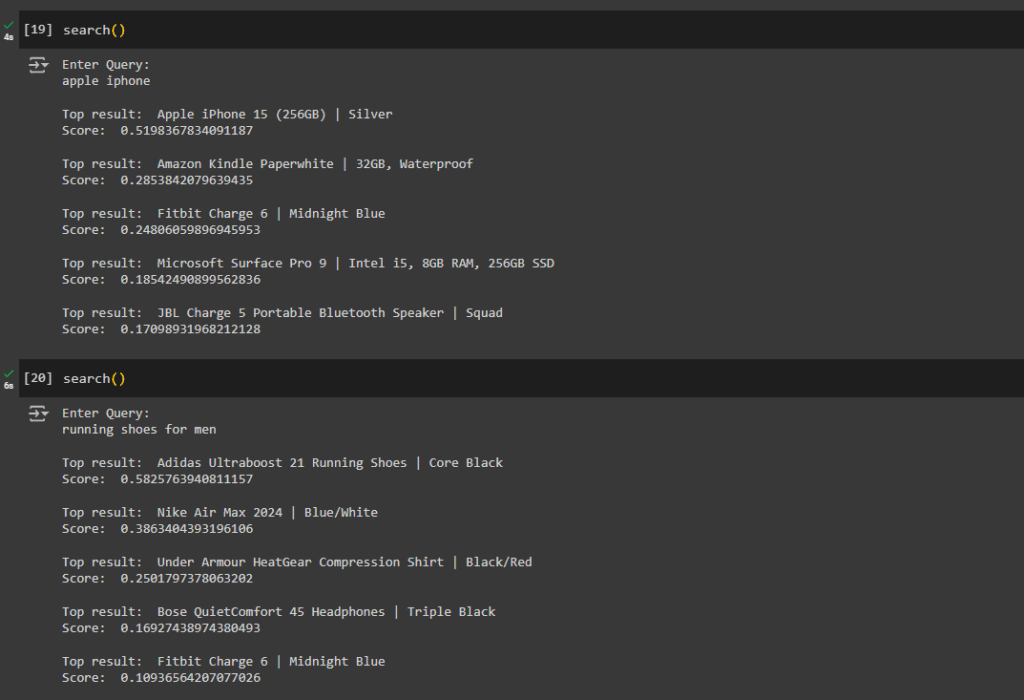
You can observe that our model is performing exceptionally well, with satisfactory scores. To further enhance result relevance, consider adding a threshold ratio of 0.5.
Conclusion
Using SentenceTransformer 3.0.0 makes training or fine-tuning embedding models a breeze. The new version boasts support for multi-GPU utilization via the DDP method and introduces logging and experimentation features through Weights & Biases. By encapsulating our code within a single main function and executing it with a single command, developers can streamline their workflow significantly.
The Evaluator functionality aids in evaluating models during the training phase, catering to defined tasks like Embedding Similarity Search in our scenario. Upon loading the model for inference, it delivers as anticipated, yielding a satisfactory similarity score.
This process harnesses the potential of vector embeddings to enhance search results, leveraging user queries and database interactions effectively.